Selection bias happens when the participants of a study don't represent the whole population you're trying to understand. This leads to errors and false conclusions, erasing your data's accuracy and reliability.
Imagine you're analyzing user behavior to improve a product but only look at responses from your most active users. Your results will be skewed, showing more engagement than what actually exists across your entire user base.
Spotting and correcting selection bias is essential for anyone involved in data analysis and product development. Ensuring your data accurately reflects your target population makes your findings reliable and valid, which is crucial for making data-driven decisions.
Quick takeaways
Selection bias happens when the participants of a study don't represent the whole population, leading to skewed data and unreliable conclusions.
To design surveys that minimize bias, use inclusive language, frame questions neutrally, and conduct pre-tests with diverse samples to ensure your surveys accurately reflect your target audience.
Regularly reviewing and validating your data collection process with methods like regression analysis, propensity score matching, and cross-validation helps identify and correct potential biases, leading to more accurate and trustworthy results.
What is selection bias?
Selection bias is a systematic error that happens when the people studied differ from the population you aim to understand, whether because they weren’t randomly picked or didn’t all stay in the study, skewing any conclusions you draw.
Video transcription:
A good example would be:
Your boss walks up to you and says, “Hey, run me some numbers and see what the growth is between the first and second week of February.”.
Think about what happens in the second week of February. It's Valentine's Day. We are all frantically buying stuff for our loved ones.
As a result, if you're in a retail world—a flower business, for example, and you are comparing the first week of data to your second week of February data—you've introduced bias in your analysis.
Or, say you had a product release on the 10th of February. You are reporting a 20% growth, but obviously, that week was going to drive a lot of volume, irrespective of what you do. These are the traps that you've got to watch, especially when you have new product releases, right? Because your data that you have for the first week versus the second week has some bias in it.
-Milin Shah, Director of Data Strategy and MarTech Enablement at UPS
7 types of selection bias
Selection bias can appear in several forms, each affecting your data differently:
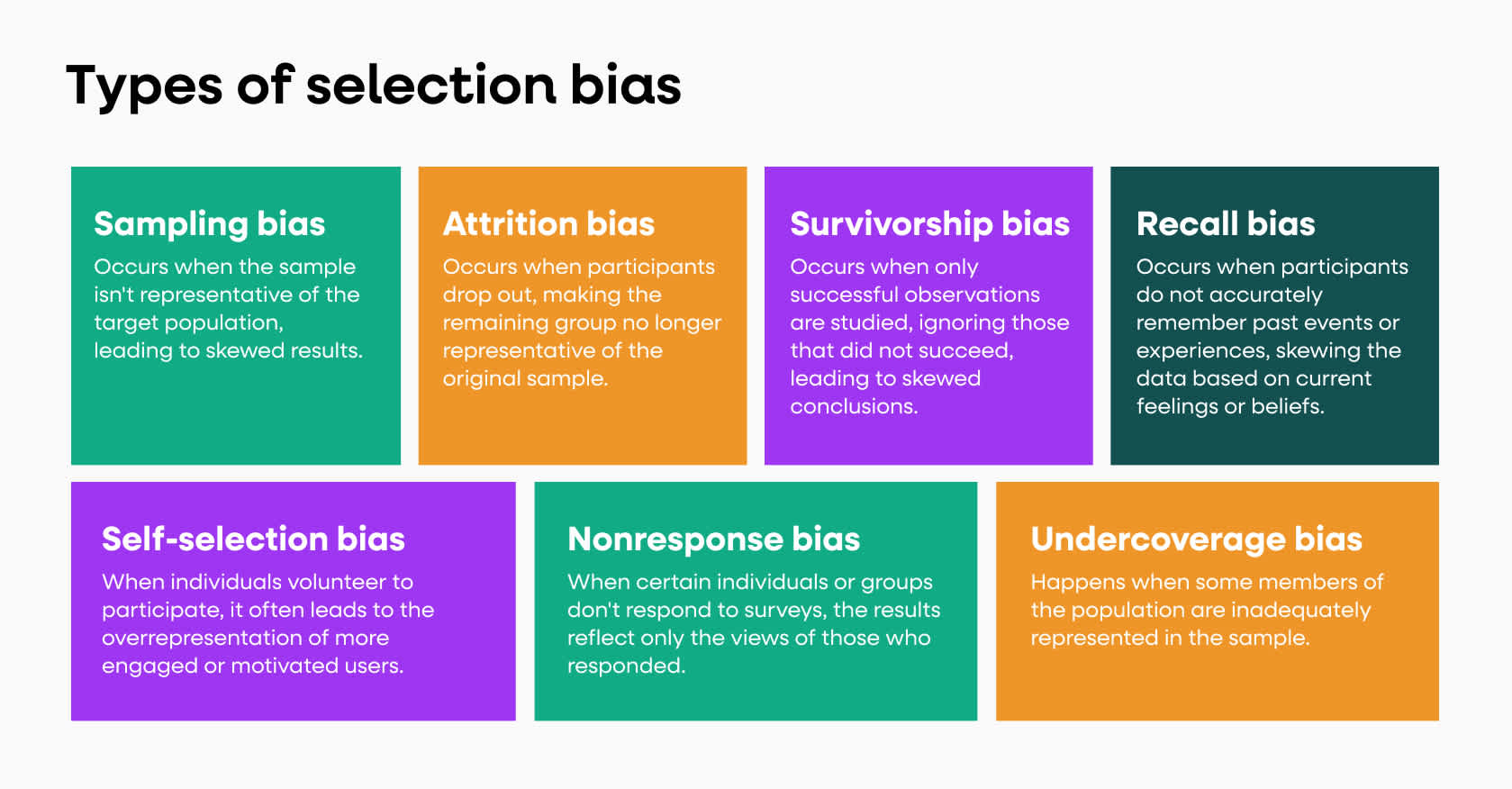
1. Sampling bias
This occurs when the sample isn't representative of the target population.
Example: If you're surveying only premium users about their experience, the insights won't reflect the opinions of lower tier users.
2. Self-selection bias
This happens when individuals volunteer to participate, potentially differing significantly from those who don't. This often leads to overrepresenting more engaged or motivated users, skewing the data.
Example: In a survey about exercise habits, those who choose to participate might be more health-conscious than the general population, leading to overestimated fitness levels.
3. Survivorship bias
This is seen when only those who "survived" a process are studied, ignoring those who didn’t make it.
Example: Analyzing only the success stories of a product without considering users who abandoned it early on gives an overly positive view of the product's performance.
4. Attrition bias
This arises when participants drop out, and the remaining group is no longer representative.
Example: If less satisfied users are more likely to stop responding to follow-up surveys, the feedback will be overly positive.
5. Undercoverage bias
This occurs when some members of the intended population are inadequately represented in the sample.
Example: If a survey about mobile app usage excludes older adults, the results won't capture their user behavior.
6. Nonresponse bias
This happens when certain individuals or groups don't respond to surveys or data collection efforts, leading to results that only reflect the views of those who did respond.
Example: A customer satisfaction survey might miss the views of dissatisfied customers who choose not to respond, skewing the results positively.
7. Recall bias
This arises when participants do not accurately remember past events or experiences, often skewing the data based on their current feelings or beliefs.
Example: In surveys asking about past medical history, participants might forget or misreport their past illnesses, affecting the accuracy of the data.
Understanding these types of bias is crucial for product and data teams to ensure their analyses reflect the entire user base accurately. Ignoring these biases can lead to product features that only cater to a segment of your users, missing broader needs and opportunities.
Identifying selection bias in research
In research, it's important to scrutinize the methods used to select participants. Ask yourself:
Were participants chosen randomly, or was there a non-random selection process?
Does the sample accurately represent the target population in terms of demographics, behavior, and other relevant factors?
Using study designs like cohort studies or case-control studies can help minimize bias. Ensure your sample size is adequate and covers all relevant demographics to avoid underrepresentation. If you're conducting a user experience study, make sure to include users from different age groups, geographic locations, and levels of engagement with your product.
Identifying selection bias in data analysis
When analyzing data, selection bias can distort your findings. Here are some steps to identify and address this bias in your data analysis:
Examine data sources: Ensure that your data sources are comprehensive and representative of your study population. For instance, if you're analyzing customer feedback, include data from various channels like surveys, social media, and support tickets.
Analyze participation patterns: Look for patterns in who is participating and who isn't. Are certain user groups underrepresented in your data? This can help you understand potential biases in your analysis.
Compare characteristics: Compare the characteristics of participants versus non-participants. If there's a significant difference, your sample may be biased. For example, if a significant portion of feedback comes from power users, the data may not reflect the experiences of casual users.
Use statistical techniques: Employ statistical methods like regression analysis to control for variables that could introduce bias. Propensity score matching can also help ensure that your comparisons are between similar groups, reducing the impact of selection bias.
Validation checks: Perform validation checks to compare included versus excluded data. This might involve looking at demographic data, usage patterns, or other relevant metrics to ensure your sample is representative.
By actively identifying and addressing selection bias, you can improve the accuracy of your data analysis, leading to more reliable insights and better decision-making.
Mitigating selection bias
Mitigating selection bias is crucial for ensuring that your research and data analysis produce accurate and reliable results. Here are some strategies to help you avoid selection bias at different stages of your study.
How to avoid selection bias
To avoid selection bias, it’s important to plan and execute your research design carefully. This involves considering how participants are selected and ensuring that your sample is representative of the entire population you wish to study. Here are key steps to avoid selection bias:
Survey design
Designing surveys to minimize bias involves crafting questions that are clear, neutral, and accessible to all potential respondents. Consider the following tips:
Inclusive language: Use language that is understandable to a broad audience and avoid jargon that might exclude some respondents.
Balanced questions: Frame questions in a neutral way to avoid leading respondents toward a particular answer.
Pre-Testing: Conduct pilot tests with a small, diverse sample to identify and address any biases in your survey design.
Types of sampling
The type of sampling method you choose can greatly influence the representativeness of your sample. Here are some effective sampling techniques to minimize bias:
Random sampling: Ensure that every individual in the population has an equal chance of being selected. This helps create a representative sample.
Stratified sampling: Divide the population into subgroups (strata) and randomly sample from each subgroup. This ensures all relevant segments of the population are represented.
Systematic sampling: Select every “n”th individual from a list of the population. This method can be effective if the list order is random.
Review and validation
Regularly reviewing and validating your data collection process is essential for identifying and correcting potential biases. Consider these steps:
Data review: Continuously review the data as it is collected to identify any patterns or anomalies that might indicate bias.
Cross-validation: Use different methods to cross-validate your findings. For example, compare survey results with actual behavior data to check for consistency.
Peer review: Have your study design and findings reviewed by colleagues or external experts to identify potential biases and suggest improvements.
Validation techniques
Applying validation techniques helps ensure that your findings are robust and reliable. Here are some methods to validate your research:
Regression analysis
: Use regression models to control for confounding variables that might introduce bias.
Propensity Score matching: Match participants with similar characteristics to reduce bias in observational studies.
Sensitivity analysis: Test the robustness of your results by varying key assumptions or including/excluding different data points.
Weighting adjustments: Apply weights to adjust for over- or under-representing certain groups in your sample.
Implementing these strategies can significantly reduce selection bias in your research and data analysis, leading to more accurate and trustworthy results.
Wrapping up: the importance of addressing selection bias
Data bias can really throw a wrench in your research and data analysis. To avoid data bias, you must start by understanding the different types.. Taking steps to avoid data bias, designing thoughtful surveys, using the right sampling methods, and regularly reviewing and validating your process are all key to ensuring your data accurately reflects your whole audience.
For product and data teams, tackling data bias isn't just a nice-to-have, rather, it's crucial for making smart, data-driven decisions that truly meet user needs. When you actively work to reduce data bias, your findings become more solid, leading to better and fairer outcomes.
Ultimately, committing to identifying and mitigating data bias helps build trust in your data and the decisions it drives, paving the way for better products and experiences for everyone.