What if you could predict every customer's next move, anticipate their needs, and deliver exactly what they're looking for before they even ask? This is not the plot of a futuristic novel but the reality of today's competitive marketplace.
By understanding and anticipating customer preferences and actions, businesses can enhance engagement, supercharge marketing efforts, and significantly improve sales.
Machine learning is largely involved in predicting customer behavior. By analyzing patterns in past purchases and interactions, businesses can uncover valuable insights into customers' preferences and use that information for more effective marketing campaigns.
Insights enable businesses to tailor their services and products to align with their customers’ needs, ultimately driving customer loyalty.
Key takeaways
Customer behavior is the analysis of how consumers choose and use products, influenced by cultural, social, personal, and psychological factors.
Businesses use predictive behavioral analytics and market research to understand and predict these behaviors to improve marketing strategies and customer satisfaction.
By utilizing these insights, businesses can improve their marketing strategies and enhance customer loyalty.
What is customer behavior?
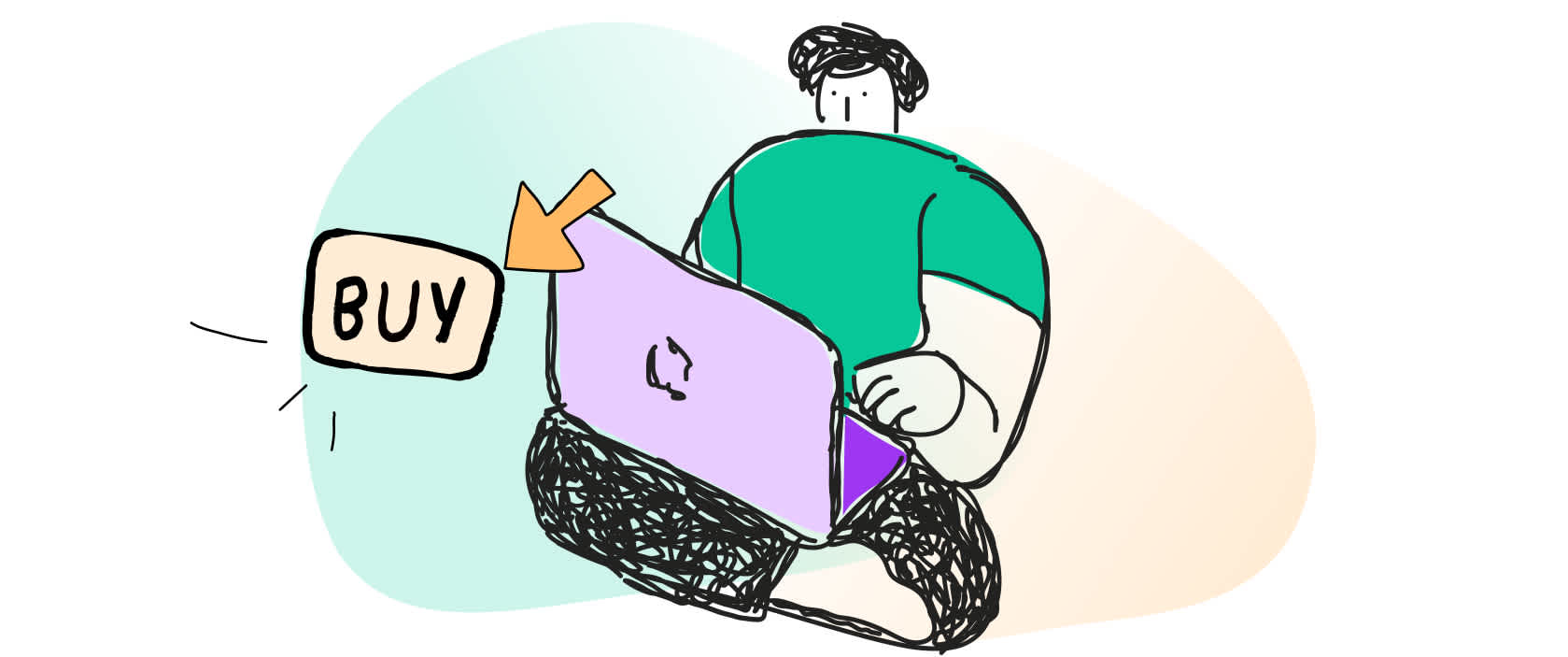
Customer behavior is the study of how people make decisions and take actions related to their consumption of products or services. It aims to understand the various patterns and trends that emerge as individuals interact with the market and make purchasing choices.
At its core, customer behavior involves researching and analyzing consumers' actions to gain insights into their preferences, needs, and desires. Businesses can then use this information to design effective marketing approaches, improve customer experience, and drive sales.
Businesses often turn to various research methodologies to analyze customer behavior effectively. These may include quantitative approaches like surveys and data analysis and qualitative methods like interviews and focus groups.
By gathering and analyzing data, companies can create more targeted marketing campaigns, develop products that meet consumer needs, and predict future behaviors.
Factors that influence customer behavior
Now that we've discussed the basics of customer behavior, you might be asking: What actually influences these behaviors?
Let's explore the diverse factors shaping consumers' decisions.
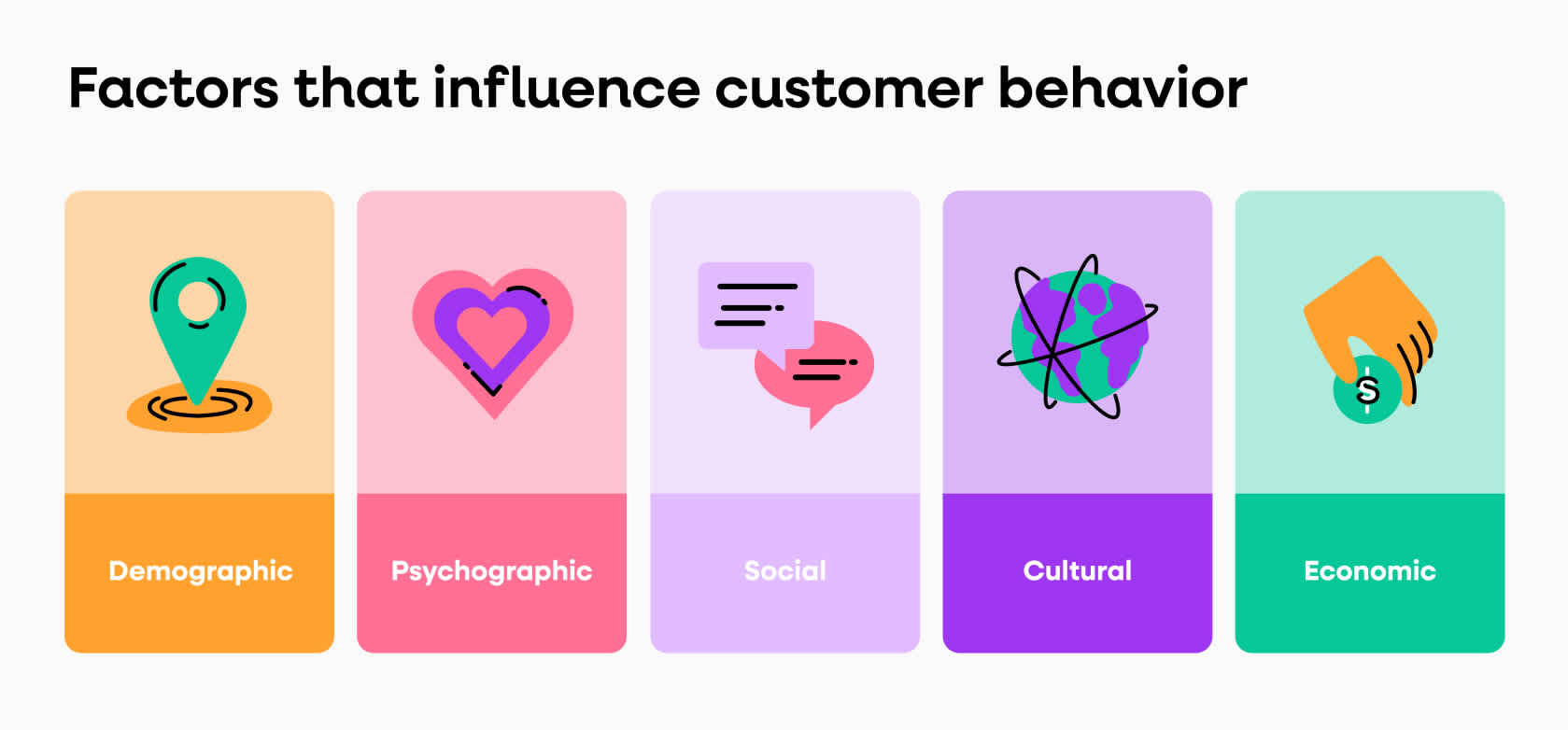
Demographic factors
Demographic factors are pivotal in grasping customer behavior, where aspects such as age, gender, occupation, and education deeply impact customers' purchasing decisions.
For example, a person's income and financial status can dictate their spending habits and preferences.
Psychographic factors
Psychographic factors explore an individual's personality, values, interests, and lifestyle, which are crucial in determining their preferred products or services.
For example, a person's commitment to environmental sustainability might lead them to favor eco-friendly brands.
Social factors
Social factors significantly influence customer behavior. The views and actions of family, friends, social media, and the wider society can shape how decisions are made.
For instance, social media trends can drive consumer interest in specific products or brands.
Cultural factors
Culture, including language, values, norms, and traditions, plays a key role in consumer behavior. People from different backgrounds may have unique preferences influenced by their cultural upbringing.
For example, dietary preferences influenced by cultural practices can determine food product choices.
Economic factors
Economic conditions are critical in affecting customer behavior. Factors such as individual income, the overall economy's health, inflation, and taxation influence spending habits and product preferences.
For example, economic growth can lead to increased spending on non-essential items, while economic recessions might see a rise in demand for basic necessities.
Types of customer behavior
When it comes to what consumers decide to buy, a lot hinges on the product or service itself. Factors like price, how easy a product or service is to get, and how often they buy it matter.
And here's the thing: how much shoppers get involved in buying depends on these factors. Also, how risky the purchase feels plays a big part.
Generally, the more something costs, the bigger the perceived risk, and the more thought and effort people put into deciding whether to buy it. This careful consideration directly influences their buying behavior.
There are 4 main types of customer behavior:
1. Complex buying behavior
Complex buying behavior occurs when customers invest significant time and effort in evaluating products before making a purchase. High-involvement products, such as cars or expensive electronics, often trigger this type of behavior.
Consumers engage in extensive research, comparing product features, prices, and reviews to ensure they make the best decision. Companies can leverage this understanding by focusing on providing detailed product information and emphasizing how their offering stands out against the competition.
2. Dissonance-reducing buying behavior
Dissonance-reducing buying behavior takes place when customers experience post-purchase anxiety or uncertainty about their decision. This can arise when consumers feel that they had to make a decision quickly, without sufficient time to weigh the pros and cons, or if their choice was informed by limited information.
To minimize such dissonance, marketing efforts should emphasize elements of reassurance. For example, companies can:
Offer clear return and exchange policies
Display positive customer reviews
Provide easily accessible customer support or AI support
3. Habitual buying behavior
Habitual buying behavior is characterized by consumers relying on routines and habits when making purchasing decisions. This type of behavior is commonly found in less involving product categories, such as groceries or personal care items, where consumers are not as inclined to research products extensively before purchase.
Consequently, companies should focus on building brand recognition and loyalty and creating memorable advertising to entice customers.
Some strategies include:
Consistent branding and packaging
Implementing loyalty programs or offering regular discounts
Utilizing eye-catching promotion strategies
4. Variety-seeking buying behavior
Variety-seeking buying behavior arises when customers actively seek new experiences, products, or brands, even if satisfied with their current choices. This behavior typically occurs in categories where products are low-involvement, low-cost commodities, and consumers feel minimal risk in trying new options.
Companies should capitalize on this by frequently introducing variations or limited-time offers. Techniques to engage variety-seeking consumers can be:
Launching seasonal or unique-flavored products
Rotating promotional offers
Collaborating with other brands for co-branded products
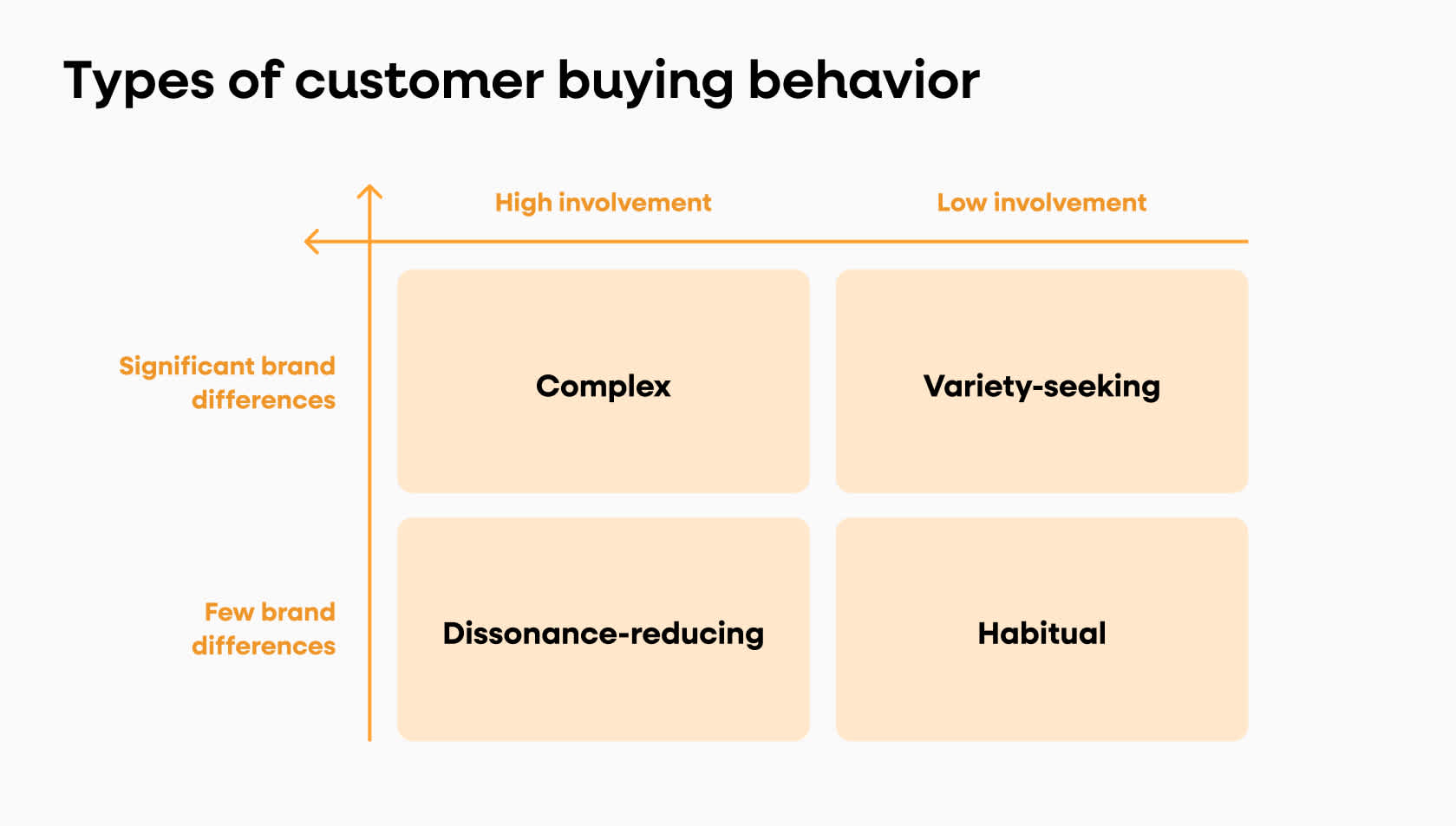
How to predict customer behavior
Understanding what drives consumer choices is essential for business success.
Let's explore three key methodologies that help predict customer behavior: data insights, market insights, and customer segmentation. Each offers valuable tools for businesses to anticipate consumer needs and refine their strategies.
Data insights
Data plays a crucial role in predicting customer behavior. By collecting and analyzing data from various sources, businesses can gain valuable insights into their customers' preferences, habits, and motivations.
These insights can be derived from online user behavior, purchase history, and demographic information. Utilizing advanced tools such as predictive analytics and machine learning algorithms can help businesses draw valuable conclusions from their data sources.
Market insights
Understanding the market trends and overall industry landscape is essential for accurately predicting customer behavior. Gaining market insights can help businesses identify external factors that may influence their customers' decision-making process.
This can include conducting market research and keeping up-to-date with the latest industry developments, competitor insights, and customer reviews. Market insights can be combined with behavioral data analytics to create a more comprehensive representation of customer behavior.
Customer segmentation
Identifying and segmenting different customer groups based on their preferences, behaviors, and motivations is an essential component in predicting customer behavior.
Customer segmentation allows businesses to better target their marketing efforts, improve customer experience, and maximize the effectiveness of their resources. Segmenting customers enables personalized predictions and tailored approaches for each group, improving overall customer satisfaction.
What is predictive behavior modeling?
Predictive behavior modeling is a technique that uses data analysis and machine learning algorithms to predict future customer behavior. It plays a crucial role in industries such as retail, finance, and marketing, helping businesses better understand their customers and user groups.
Companies collect large amounts of behavioral data from various sources, such as transaction histories, browsing patterns, and customer interactions, to create a predictive model. The data is then processed, cleaned, and analyzed to identify trends and patterns that can be mapped to future behavior. Some common applications of predictive behavior modeling include:
Estimating customer lifetime value
Identifying potential churn risks
Discovering cross-selling and upselling opportunities
Forecasting revenue and sales
Using machine learning algorithms like decision trees, k-means clustering, and neural networks, these models grow more accurate over time as more data is added.
Although predictive modeling provides significant insights, it’s important to use it as a tool to enhance, rather than replace, human intuition. When used correctly, it helps companies design targeted promotions, improve customer retention, and increase revenue by effectively predicting and meeting customer needs.
How machine learning can predict customer behavior
Machine learning has become an essential tool for predicting and understanding customer behavior. It allows businesses to anticipate customer needs and preferences, leading to improved customer engagement and retention.
Now, let's explore how machine learning can be utilized in various aspects of customer behavior prediction.
Personalize campaigns
Machine learning can identify patterns and trends that help businesses create tailored marketing campaigns by analyzing historical customer data. By tailoring product recommendations, promotional offers, and advertisements, companies can engage customers more effectively, leading to increased loyalty and a better shopping experience.
For instance, advanced marketing analytics can predict cross-selling opportunities or the likelihood that a customer will be interested in purchasing additional products or services. The result is highly targeted efforts that resonate well with customers.
Improve customer satisfaction
Machine learning can improve customer satisfaction by identifying and addressing potential customer pain points. By predicting common customer complaints, businesses can take prompt action to rectify the problems before they escalate, leading to higher levels of customer satisfaction.
For example, Complaints Management can anticipate customer grievances, helping companies address these issues proactively.
Predict customer churn
Determining when a customer might leave a company is vital for customer retention. Machine learning algorithms can analyze vast amounts of data to understand patterns in customer churn. Using these predictive insights, businesses can devise tailored strategies aimed at keeping customers engaged and loyal.
For instance, by identifying customers who are at a higher risk of churning, companies can offer targeted promotions or personalized solutions to retain their patronage. Implementing this ensures long-term customer loyalty and improved revenue streams.
Detect fraud
Through user behavior analytics tools, machine learning can also help detect potential fraud cases.
Suspicious transactions, account creations, and other activity can be flagged to prevent losses and protect legitimate customers. The machine learning algorithms sift through vast amounts of data, quickly identifying potential risks and allowing businesses to conduct early investigations.
A future shaped by customer behavior
Predicting customer behavior is a game-changer in any business plan. Data science and predictive analytics can help keep customers, make them happy, and push sales up. But it's not just about having the tools; understanding your business, who your customers are, and what your data tells you is just as important.
Using machine learning and constantly refining your data and models can shed light on how your customers behave. When you get it right, predictive analytics can take your marketing to the next level, keep your customers coming back, and help your business grow.
The effort required to tailor these techniques to your specific needs is well worth it, as they promise great results for your company's journey.
Want to know where your company stands with behavioral data? Download the Fullstory Data Maturity Matrix and take our quiz.